- The UDA framework emphasizes the need to build an appropriate acoustic capacity to lend a hand in underwater conservation and sustainable growth.
- The composition of the ocean soundscape has drastically altered post-industrial revolution due to the ramping up of anthropogenic activities in the ocean that disturb the natural functioning of marine species.
- The first step towards recognizing the potential areas for improvement is through recording and accessing the composition of marine soundscapes to better interpret the effects caused by abiotic sources.
- Soundscape monitoring is gradually gaining significance in designing more informed and effective managerial policies. This has the potential to pave the way for a protected acoustic ocean environment in the future.
Heading
Sound plays an inevitable role in the lives of ocean-dwelling species. It travels the fastest and farthest in the ocean. Marine species from marine invertebrates to large-sized cetaceans rely on sound for their survival. Its unique adaptations aid these species to communicate, navigate, localize, and understand their ambient environment. A soundscape is a combination of sounds that arises from an immersive environment. Ocean soundscapes are made up of sounds produced by anthrophony (ocean-based anthropogenic activities such as shipping, fishing activities, dredging, exploratory and recreational activities), biophony (marine species such as marine mammals, fishes, and marine invertebrates) and geophony (ocean-based geophysical activities such as rain dropping hitting the ocean floor, gushing waves, iceberg breaking and undersea earthquakes, volcanic eruptions). The composition of the ocean soundscape has drastically altered post-industrial revolution due to the ramping up of anthropogenic activities in the ocean that disturb the natural functioning of the marine species, hindering their communication largely. The current ocean soundscape shows a decreased abundance of marine species, rising anthropogenic activities and the erratically occurring geophysical activities. The ambient ocean noise from the anthropogenic sources is found to have a masking effect on the marine species’ auditory system that weakens their ability to forage for prey, escape from predators or attract their mate. Their prolonged effect, in the long run, has notably diminished the population of certain marine species.
Ocean acoustics, the propagation or behaviour of sound underwater forms the basis for communication in oceans. Sound as signals from diverse ocean sources constitute the ocean’s ambient noise. This sound when perceived as noise possesses major ill-effects that benefit. Increasing ocean noise has become an invisible threat to marine life and its intensity is observed to have grown bounds ever since the industrial revolution. This scenario has created mounting pressure on the ocean life, affecting their survival. This has been acknowledged by scientists, who observed ocean soundscapes quieted by COVID-19, with human activities in the oceans being completely shut and noted a positive behavioural change in the marine life. Findings as such support the cause for better policies to control anthropogenic activities in the ocean for the protection of marine life.
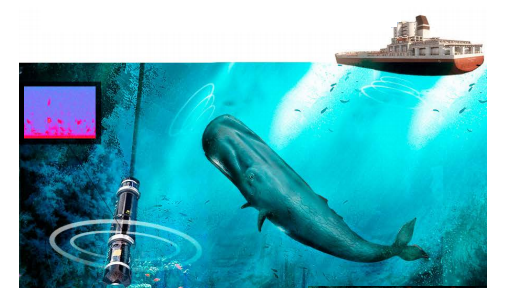
However, the first step towards recognizing the potential areas for improvement is through recording and accessing the composition of marine soundscapes to better interpret the effects caused by abiotic sources. Given that sound travels faster and deeper in the oceans, an underwater microphone called the hydrophone is used to record the ocean noises using a non-invasive technique called Passive acoustic Monitoring (PAM). Owing to the inaccessibility of deep underwater regions, the hydrophones are deployed for a relatively long time period leading to recording larger volumes of passive acoustic soundscape recordings. Previously, methods like visual surveys and invasive methods such as traps and trawls were largely employed to document the presence and diversity of underwater species. However, their time and resource-intensive nature lead to the difficulty in capturing long-term trends in certain protected areas. These recordings form as the basis for characterizing the ocean soundscape by observing the variability and changes in the ocean noise over time. Addressing the issues involved in interpreting the recorded soundscape data demands an understanding of the composition of the soundscape under study which is achieved by separating the individual sources from the real-time raw passive acoustic recordings using sound source separation techniques.
Soundscape source separation and its role in marine biodiversity conservation
Soundscape source separation or soundscape information retrieval is a technique to recover and reconstruct individual sound sources from a mixture of sources of an ocean soundscape. However, the multiple interfering sources in a marine environment hinder the extraction of source-specific information from the biophony from the other interfering sources of geophony and anthrophony. The overlapping sources with a single biological source producing varied calls leads to biased measurements due to signal distortions.
Machine learning in Soundscape source separation
In recent decades, observing the activities of vocalizing animals underwater using tedious manual inspection methods is slowly being replaced with automated systems for performing tasks like separation. In this context, machine learning (ML) plays a major role in separating the acoustic sources without the need for an extensive recognition database. “Data” plays a major role in the design of a machine learning model as it influences the model’s accuracy largely. Adding to the importance of data, the algorithm has an upper hand in deciding the model’s performance. A perfect amalgamation of data and the algorithm constitutes machine learning and its application on a real time environmental data like ocean soundscape. The nature and the volume of the data decides the model of deployment to be either supervised or unsupervised machine learning. The performance of unsupervised machine learning largely depends on the size of the training data used. However, preparing a recognition database from the massive volumes of passive acoustic soundscape data that is recorded every year is manually tedious and are often prone to errors as their annotations are often performed by human experts. Owing to the difficulty in recording and annotating these data, their availability is relatively less compared to terrestrial soundscape data. The choice of the machine learning model to perform soundscape source separation falls under three main categories: supervised machine learning, unsupervised machine learning and semi-supervised machine learning.
Supervised learning or supervised machine learning model uses annotated data to train the model to perform tasks such as separation or prediction accurately. The model adjusts the weights based on the input data and during the cross-validation process, it ensures that it fits appropriately to avoid model over fitting or under fitting. Few popular supervised methods include neural networks, Naïve Bayes, linear regression, random forest, and many more. The unsupervised approach of machine learning uses machine learning algorithms to analyse and cluster unannotated data. These algorithms perform the discovery of hidden patterns and data grouping without human intervention. It is the most preferred choice of machine learning for exploratory data analysis, cross-selling strategies, and pattern recognition. Neural networks, k-means clustering, and probabilistic clustering methods are few unsupervised machine learning algorithms.
Effective conservatory and management efforts necessitate accessing the past, current, and future conditions of habitat in its environment. The analysis of marine soundscapes focusing on the evaluation of soundscape dynamics and the automatic identification of biotic sources for conservatory efforts rely on the capability to convert the information of various geophysical, biological, and anthropogenic events from the recorders to an automated system. Soundscape monitoring is gradually gaining significance in designing more informed and effective managerial policies which are paving the way for a protected acoustic ocean environment in the future. The development of eco-acoustic applications provides greater insights into the structure and the health of marine habitats. The ocean awaits a recovery by humans from human-caused damage. Let us protect the blue so that the green can flourish.
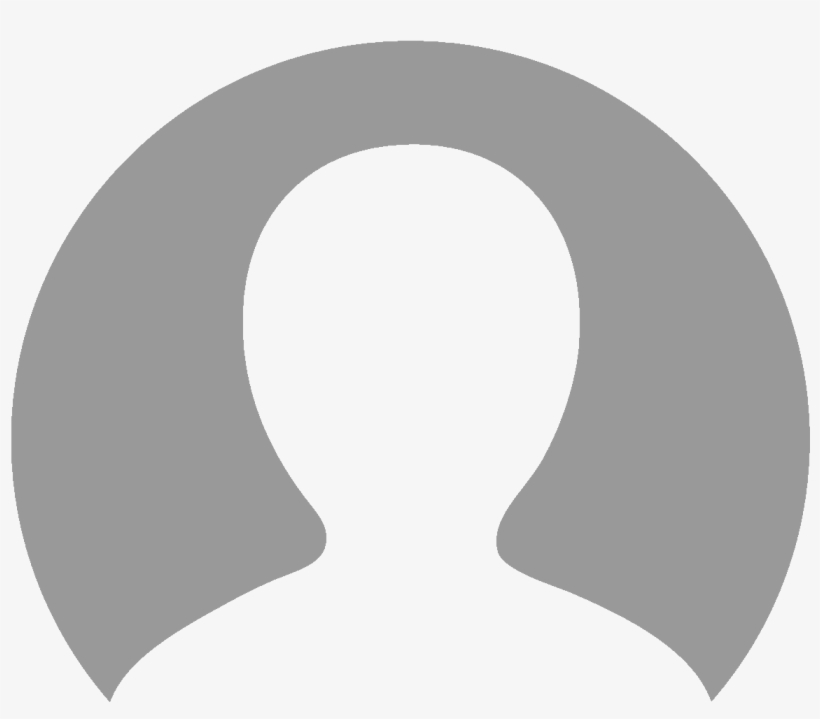
Dr. B Misha Chandar
About Author
Dr. B Misha Chandar works as an Assistant Professor VIT University- AP